Datanova 2023 | This one’s for the Data Rebels
- Monica Kay Royal
- Feb 9, 2023
- 5 min read
Updated: Apr 5, 2023
Brought to you by: Starburst
This event is for the Executives, CIOs, CDOs, Directors, Data Analysts, Data Scientists, Data Engineers, and pretty much all Data Rebels!! It is a great place to learn about treating data as a product, the ROI of data mesh, unlocking the value of distributed data, and possibly finding out a way to stop moving / copying data 😀
Why is there a space race?
The agenda includes guests from this world and beyond! No seriously, we opened Day 1 with a talk from Tara Ruttley, Chief Scientist at Orbital Reef and keynote speaker who empowers others to better adapt to any situation by helping them understand how their brains process novelty. I couldn’t think of a better way to start off an event like this as Tara flies into the topic of space.
This was a fast paced discussion all about what and who exists out there with two big takeaways:
There are differing opinions on where space begins (50 miles says NASA, but the international community says 62 miles)
There is an Outer Space Treaty that outlines things such as the use of the moon.
Fascinating!!
Tara also talks a bit about space tourism, its growing popularity, and the effects space has on your physical and mental state. Taking a trip to space is not your ordinary vacation (as you can probably imagine). There are many factors involved including gravity, radiation, temperature, and nutrition which transform you into a completely different person when you get back to Earth.
With all the knowledge and passion for space Tara shared, it was a little surprising to learn that she is actually not a Treky (a fan of Star Trek). I am now curious what her favorite sci-fi movie is, there has to be at least one 👽
The data lies (and truths)
Many of us have heard the phrase ‘the data doesn’t lie’, but is that true?
A lie gets halfway around the world before the truth has a chance to get its pants on. ~Winston Churchill
Companies and people are always trying to find the answer to their problems so they often turn to the data. But the impact of falling for lies can cause wasted time, increased complexity, increased cost, and inaccurate decisions. So what are these data lies and their truths?
Lie: You need to centralize your data
Why: This is impossible!
Truth: You need to optimize for decentralized data
Lie: The modern data stack is modern
Why: It’s the same stack from decades ago… just in the cloud
Truth: Modernization is a process, not a stack
Lie: You’re ready for the IA + ML deep end
Why: A model is only as good as the data it is trained on
Truth: You’re ready to set the foundation for AI + ML
Lie: You need to hire to close the skills gap
Why: You can’t hire or upskill your way to success
Truth: You can simplify the complex
Lie: Vendor benchmarks measure real-world performance
Why: Query speed DOES NOT EQUAL Performance
Truth: Performance is multidimensional
Resources:
Take a look at the Data Lies blog series
The future of data: A BCG study
Boston Consulting Group performed a study to find out how companies can derive the most value from an organization's single most important asset: Data. This is what they found:
Data volume and complexity continues to grow, but large volumes of data are still not fully used for analytics
There is architecture complexity and market confusion because of stack fragmentation and vendor proliferation
Technology is changing faster than customers can adopt, who are still challenged with talent and complexity
The economics are shifting, and costs are headed towards a tipping point
We are in early innings of data products or meshes, expect market shift and adopt pragmatic approach
Resources:
Teaching data engineers to be curious
This was my favorite session of the day! Richard has great presentation skills and considers himself as a Professional Screw-up. He explains that this means he is able to learn from his mistakes, and does not fear failure. He also mentions that he is pretty good at not breaking things, but only by improvement to his performance through consistent trial and error. The thing that helped him with this improvement is that he is always curious to find a solution to the problem!
In order to successfully solve problem, we must not only seek help, but also ACTIVELY foster an environment to instill curiosity in others
How to instill curiosity?
It’s not all that difficult really. According to cognitive scientists and researcher Elizabeth Bonawitz, curiosity is innate in all humans. The biggest takeaway here is to foster an environment for curiosity.
Good thing Richard shared these tips:
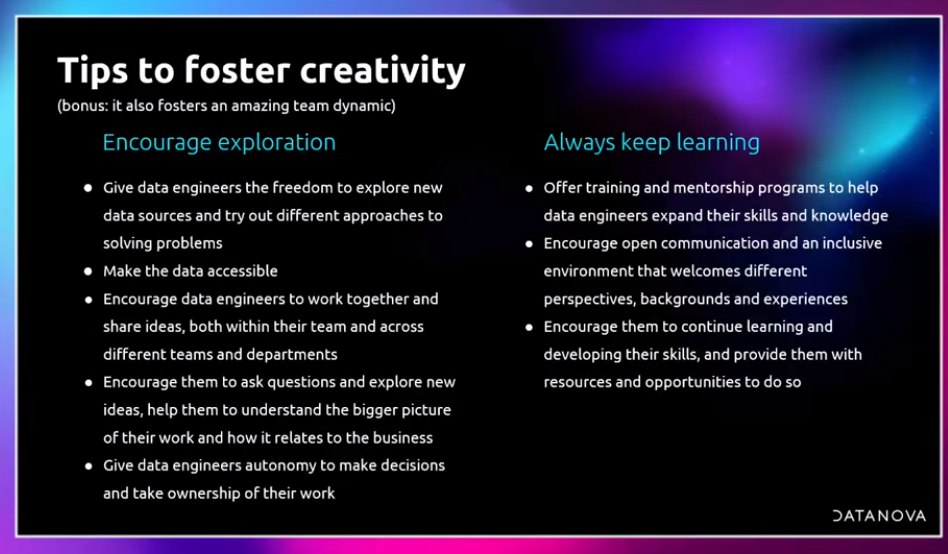
Lastly, it is important to remember that curiosity doesn’t grow successfully overnight #alwayskeeplearning
Is data mesh the end of data engineering?
Joe Reis, best-selling author of Fundamentals of Data Engineering, along with Starburst technical experts, Colleen Tartow and Andy Mott, participated in discussion on whether the adoption of Data Mesh creates opportunity or spells doom for the field of Data Engineering.
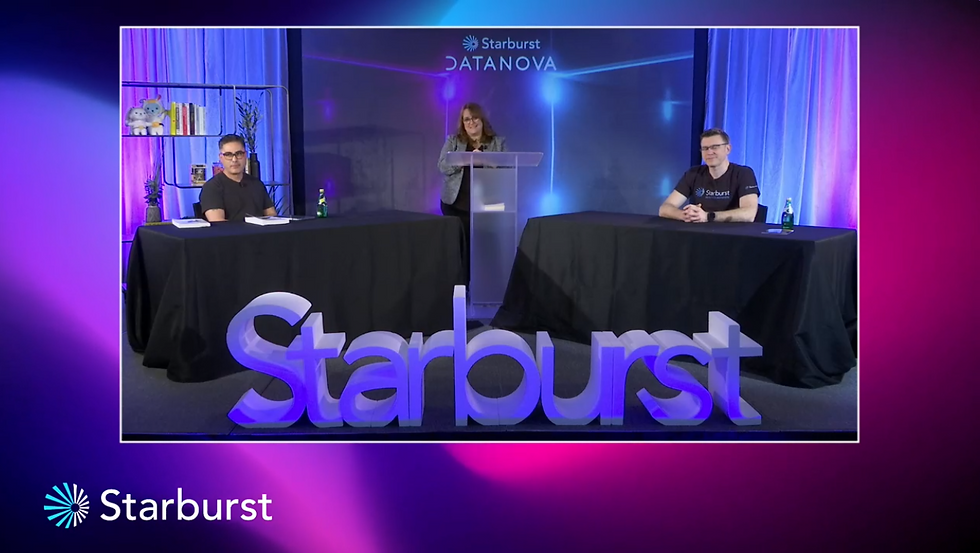
While some viewed this as an Oxford style debate, others felt like they were watching Between Two Ferns.
Nevertheless, this was a fun way to start the day!
Joe thinks that Data Mesh is the end of Data Engineering, while Andy thinks that there is a place for Data Engineering within a Data Mesh. Joe and Andy start off on opposing sides but are they able to hash things out and get on the same page in the end?
This may be difficult since Joe is a best-selling author and Andy only does as little as he can at Starburst, but did manage to help write a small pamphlet.
Throughout the entire debate Joe and Andy could not agree on anything from the definition of a Data Engineer to if humans have empathy. Joe even fell asleep while Andy was describing the 3 different models in which a Data Engineer can work within an organization. Just when they started to come to an agreement with the topic of Data Modeling, it was ruined by Joe’s overuse and insertion of the word ‘Product’ into the Data Engineer title.
Resources:
To keep the discussion going, you can sign up for the Data Mesh Book Club
An introduction to data contracts
If you are fairly new to data contracts, like myself, this was a great introduction!! I am not going to dive into detail here, but this is what I gathered:
Goal
To provide accountability and help drive great conversations and collaboration between Data Producers and Data Consumers
or as Monica Miller phrased it - To avoid the ‘kicking it down the line’ problem
What
Data Contracts are basically an API for what the data is and includes things such as the data schema, attributes, KPIs, and SLAs.
Where
There are 2 data environments
A prototype environment used for building things quickly and testing them out
A production environment used for your production products.
Production Environments should require contracts, but not every pipeline needs a contract!
When
If you know that data will be an issue down the line (this provides enough foundation to start having conversations with Data Producer teams)
When you have a data product that is actively breaking
Why
To facilitate preventative checks
Schema (define and perform checks during phases like CI/CD)
Value Constraints (IDs that should be 10 characters are actually 10 characters)
Semantics (relationships from one data object to another data object)
Make these checks as close to the source system, push accountability to the Data Producer!
Fixing data quality issues at the source!!
Resources:
Follow Chad on substack
Check out Chad's Data Quality Camp
Conclusion
Starburst | Datanova did it again, bringing together the best professionals to talk about all the emerging data and technologies from out of this world! 👽 Until next time...
Thank you for reading, Thank you for supporting
* links to Amazon are affiliate links, we earn a small commission for your purchase at no price difference to you
コメント